Weill Cornell Medicine scientists have developed a machine-learning algorithm capable of predicting biological targets of prospective drug molecules.
The new tool, described in a paper in Nature Communications on Nov. 19, could speed the development of new and better drugs, as well as the repurposing of existing drugs. Working with a pharmaceutical company, the scientists used the artificial intelligence (AI) tool to predict the target of a potent anticancer compound whose mechanism in cells was not previously known. This new information prompted the company to refocus clinical trials on brain cancer patients—trials that so far have resulted in long-term regressions for a significant subset of patients.
“Some patients are really benefiting from this drug, which is almost a miracle because the type of brain tumor they have is otherwise almost always incurable,” said study senior author Dr. Olivier Elemento, director of the Caryl and Israel Englander Institute for Precision Medicine, associate director of the HRH Prince Alwaleed Bin Talal Bin Abdulaziz Alsaud Institute for Computational Biomedicine and a professor of physiology and biophysics at Weill Cornell Medicine. “As far as I know this is the first time an AI system has been used this way to bring about a clinical success.”
“There are still lots of ways we can build upon this, but we’re all excited to see the initial impact it has had on clinical development,” said Dr. Neel Madhukar, who as a Weill Cornell Graduate School of Medical Sciences doctoral student in Dr. Elemento’s laboratory, and later a postdoctoral associate at Cornell Tech, was the primary developer of the new AI tool.
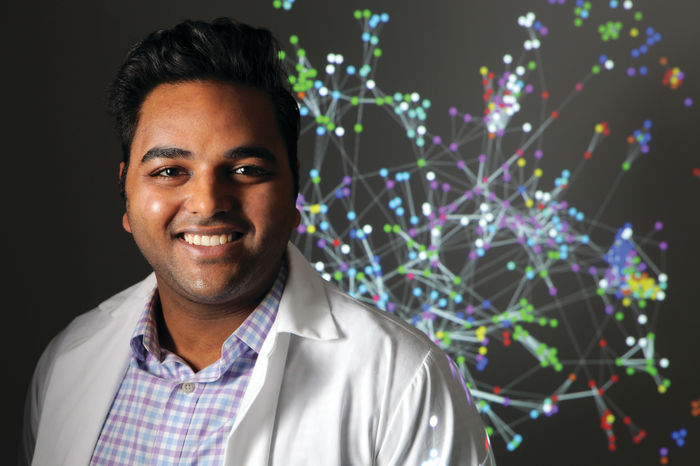
Dr. Neel Madhukar. Credit: John Abbott
Named BANDIT (Bayesian ANalysis to determine Drug Interaction Targets), the tool is a machine-learning algorithm designed to make use of virtually all available data on any prospective drug compound, in order to predict what enzyme or receptor or other target it interacts with in cells to have its therapeutic effect. Pharma chemists often screen “libraries” of thousands of small organic molecules to find some that have a desired effect on cells, but the necessary task of determining precisely how those molecules work—what targets they hit—can be highly time-consuming and labor intensive. BANDIT in principle can greatly streamline this process by narrowing the possibilities to perhaps only one or two targets that can then be investigated in depth.
Dr. Madhukar developed the BANDIT algorithm and trained it using publicly available data on more than 2,000 drug compounds. For each of these compounds, the training database contained data on the compound’s effectiveness in clinical trials, its effects on gene activity, its molecular structure, its effects on cells in lab-dish experiments, its reported adverse side effects and its known targets. The training essentially “taught” the algorithm how to predict any molecule’s target by comparing it to other molecules whose targets are known.
In one of their first demonstrations of BANDIT’s utility, the researchers collaborated with Dr. Paraskevi Giannakakou, professor of pharmacology in medicine at Weill Cornell Medicine, to find an anticancer compound that works with a unique mechanism of action to overcome tumor drug resistance.
“BANDIT identified a novel microtubule inhibitor with activity against breast cancer cells that were resistant to all clinically approved microtubule inhibitors,” said Dr. Giannakakou. “This discovery provides us with a therapeutic path forward.”
After Drs. Madhukar and Elemento began discussing BANDIT at scientific conferences, they were approached by pharmaceutical company researchers who had been developing the compound ONC201. The compound appeared to kill different types of cancer cell in lab dish experiments, but its target was unknown, leaving the company unsure about the best way to test it in clinical trials. The Weill Cornell Medicine scientists used BANDIT to predict that ONC201 hits an unexpected—and for an anticancer drug, unique—target, the dopamine D2 receptor, which is expressed by a variety of cell types but in the greatest amounts in certain brain cancer cells.
“The company then performed experiments to confirm that this receptor is indeed ONC201’s target, repositioned it as a potential treatment for brain cancers called gliomas because they express high levels of dopamine D2 receptor, and has since found that it appears to work really well in some glioma patients who no longer responded to standard treatments,” said Dr. Elemento.
Drs. Madhukar and Elemento have formed their own company to use BANDIT and other AI technologies to help drug developers find targets, and even to do their own drug development work. They are co-founders and equity stock holders of OneThreeBiotech, a company that combines biology with artificial intelligence to uncover novel biology and build the next generation of drug discovery.